Cloud Data Engineering
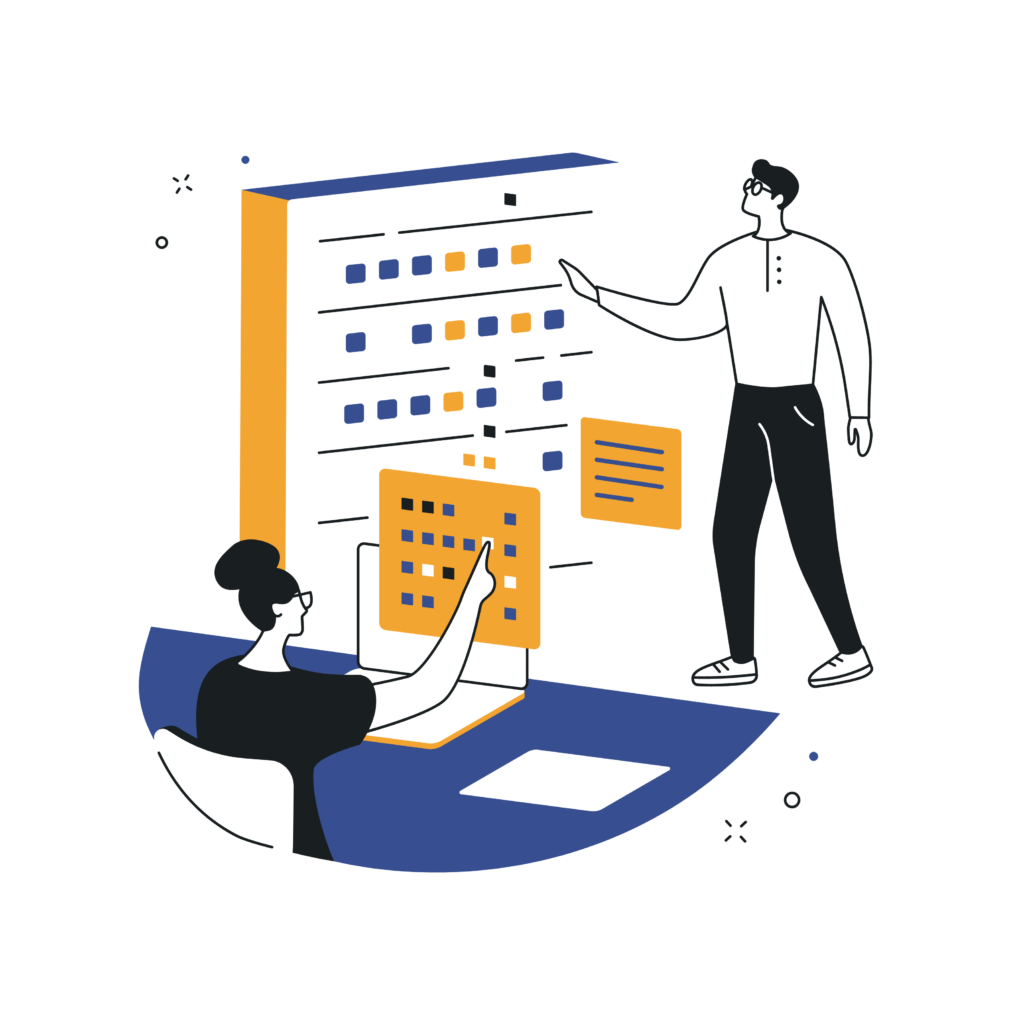
Cloud Platforms
Cloud Technologies for Data Engineering support Scalability, cost-effectiveness, flexibility and Security.
Akira provides IaaS, PaaS, SaaS Cloud solutions across cloud platforms like AWS, GCP, Microsoft Azure, Snowflake, Redshift etc. depending on the client’s requirements.
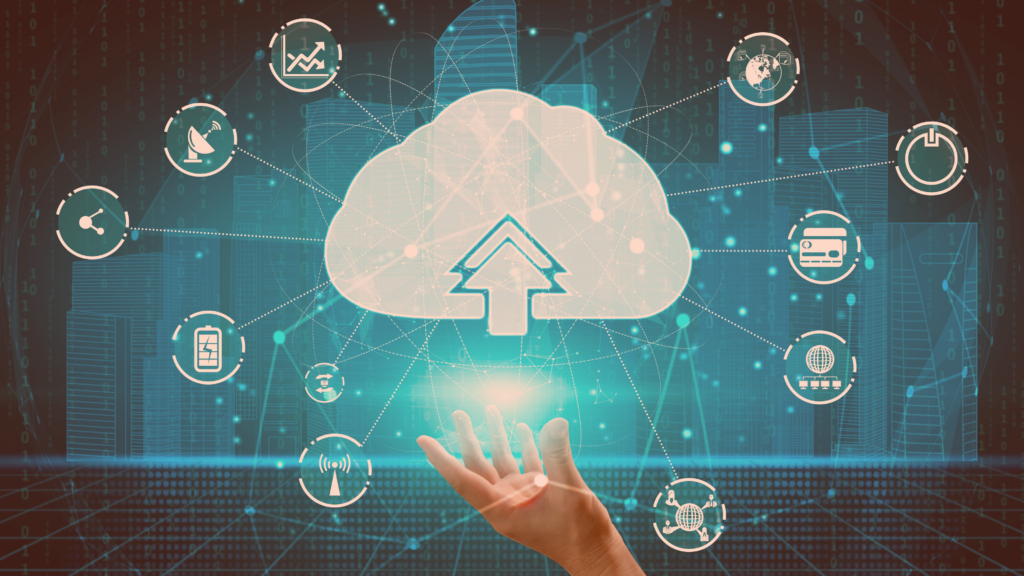
Enterprise Data Analytics Platform in Azure
Common data model, Medallion Architecture, ELT, Unified data lake and Consumption layers
EDW Architecture, Design & Implementation
DE is one of foundation pillar practices in Akira that is helping clients and customers derive solutions & insights for their business problems.
An enterprise data warehouse (EDW) is a relational data warehouse containing business data organised systematically and enables data analytics, which can inform actionable insights.
EDWs collect and aggregate data from multiple sources, acting as a repository for most or all organizational data to facilitate broad access and analysis.
Akira has created robust client-spcific EDW’s and thus, ensured that the data is consistently available on a real-time basis for analytics and sharing.
Snowflake Data Warehousing
Migration from on-premise to Snowflake and AWS 31 to 35
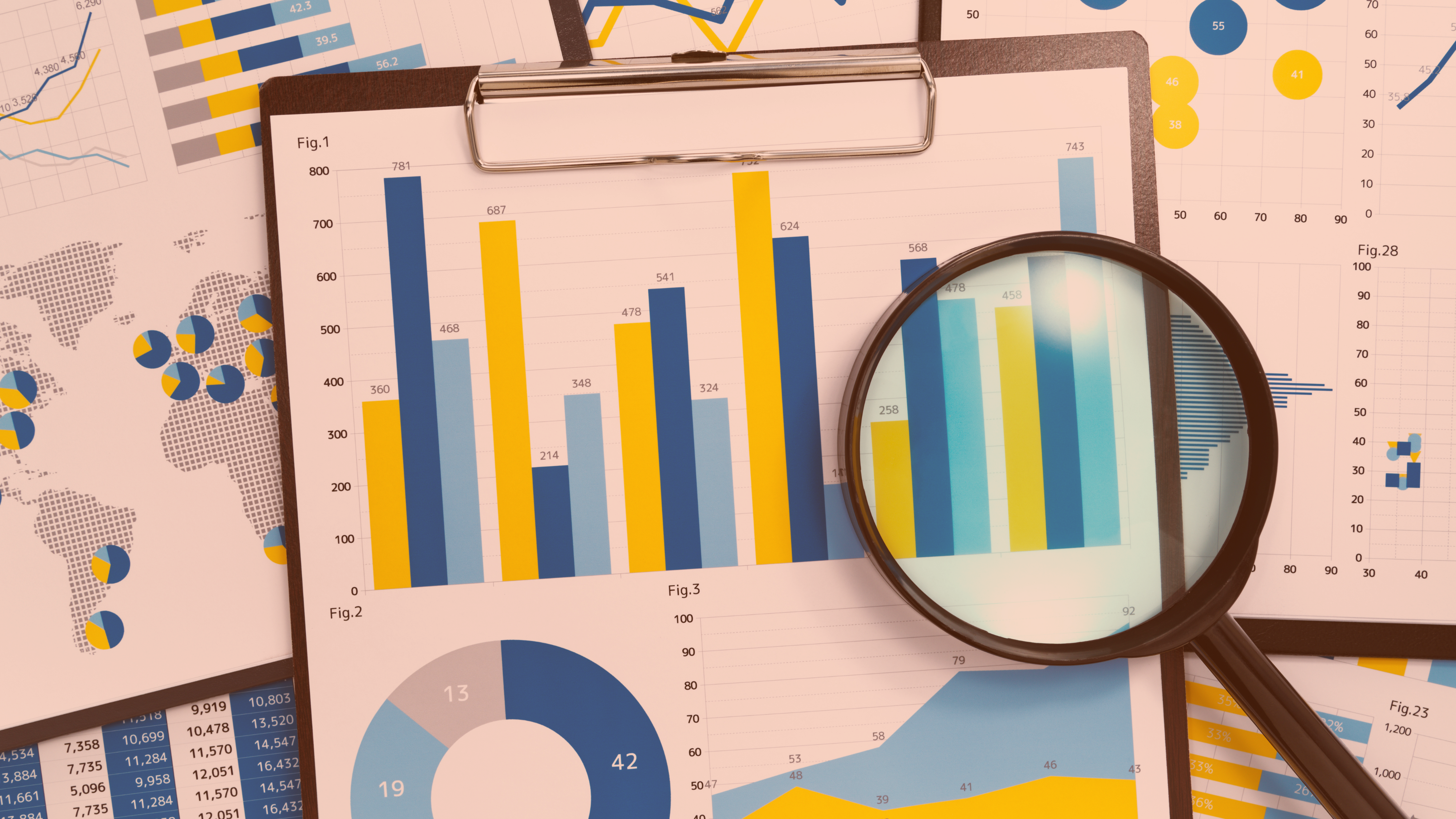
Data Lake Architecture, Design & Implementation
The aim is to host a performance-efficient & scalable platform using DE tools & technologies that deliver quick results and actionable insights also coupled with predictive & prescriptive analysis depending on client’s needs.
Data Lake is a Storage Repository that can store large amount of structured, semi-structured, and unstructured data. It is a place to store every type of data in its native format with no fixed limits on account size or file. It offers high data quantity to increase analytic performance and native integration.
Akira has facilitated design & implementation for multiple clients by sourcing data across hetergenous on-premise and cloud sources, created numerous layers to facilitate cleansing & business transformation and delivered business-ready data via Batch and real-time processes.
Skilled Data Engineers have also implemented Data Governance and Security parameters which are of prime importance to any Data Lake Architecture.
AWS Data lake & Real time Data Processing
Handling multiple sources, data integration.
Data Profiling, Quality Management
Data profiling is an assessment of data that uses a combination of tools and the purpose of data profiling is to uncover inconsistencies, inaccuracies, and missing data.
It is also be a key process of discovery for analysts to uncover the structure, content, and relationships between different data sources.
Akira ensures that standard practices are followed for Data cleansing activities and engineers have also implemented multiple processes to manage the quality of data as this is crucial for any datawarehouse implementation.
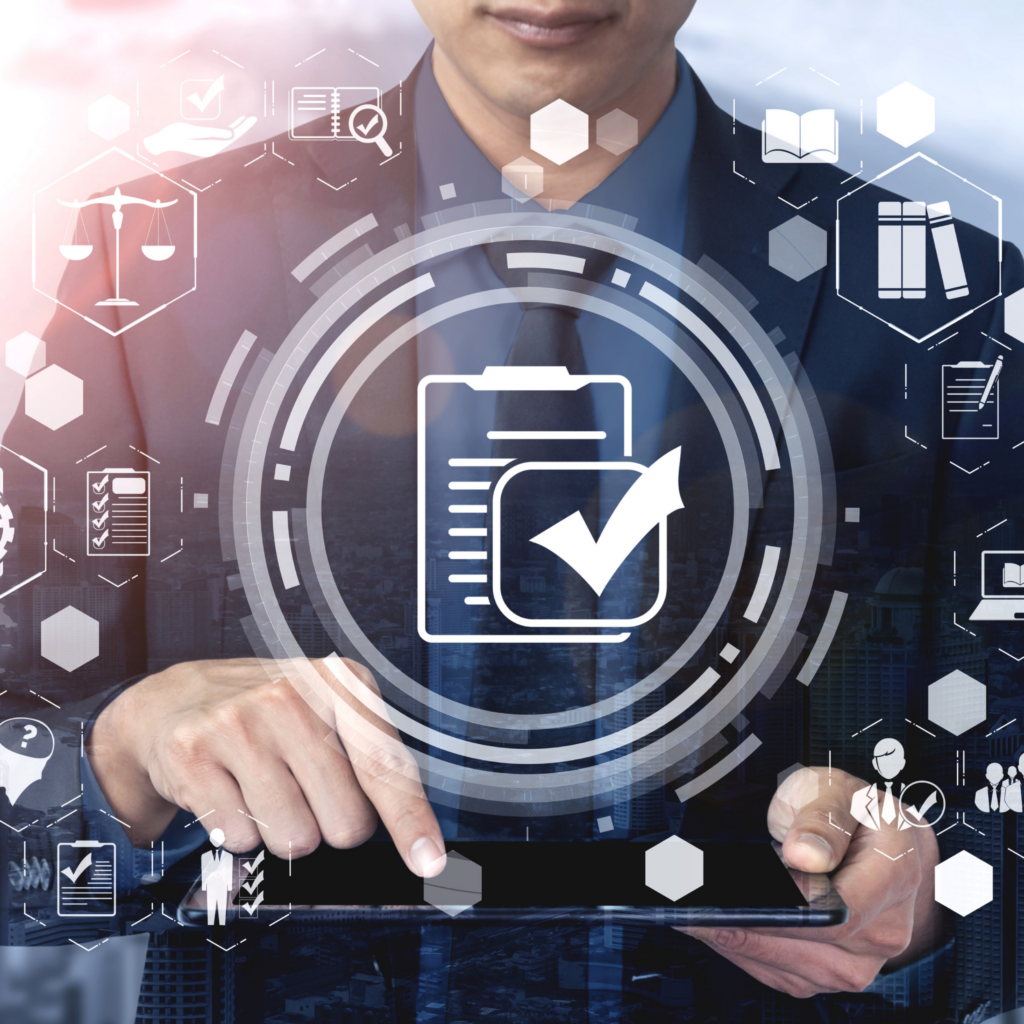
Data Mesh Implementation
Akira’s data engineers’ are experts in a wide variety of cloud platforms like AWS, Microsoft Cloud Azure, Snowflake and are leveraging their skills to meet the increased demand for faster, cheaper, and easier data storage, processing, and analysis.
Data profiling is an assessment of data that uses a combination of tools and the purpose of data profiling is to uncover inconsistencies, inaccuracies, and missing data.
The data as a product is a principle that projects a product thinking philosophy onto analytical data. Unlike traditional monolithic data infrastructures that handle the consumption, storage, transformation, and output of data in one central data lake, a data mesh supports distributed, domain-specific data consumers and views “data-as-a-product,” with each domain handling their own data pipelines.
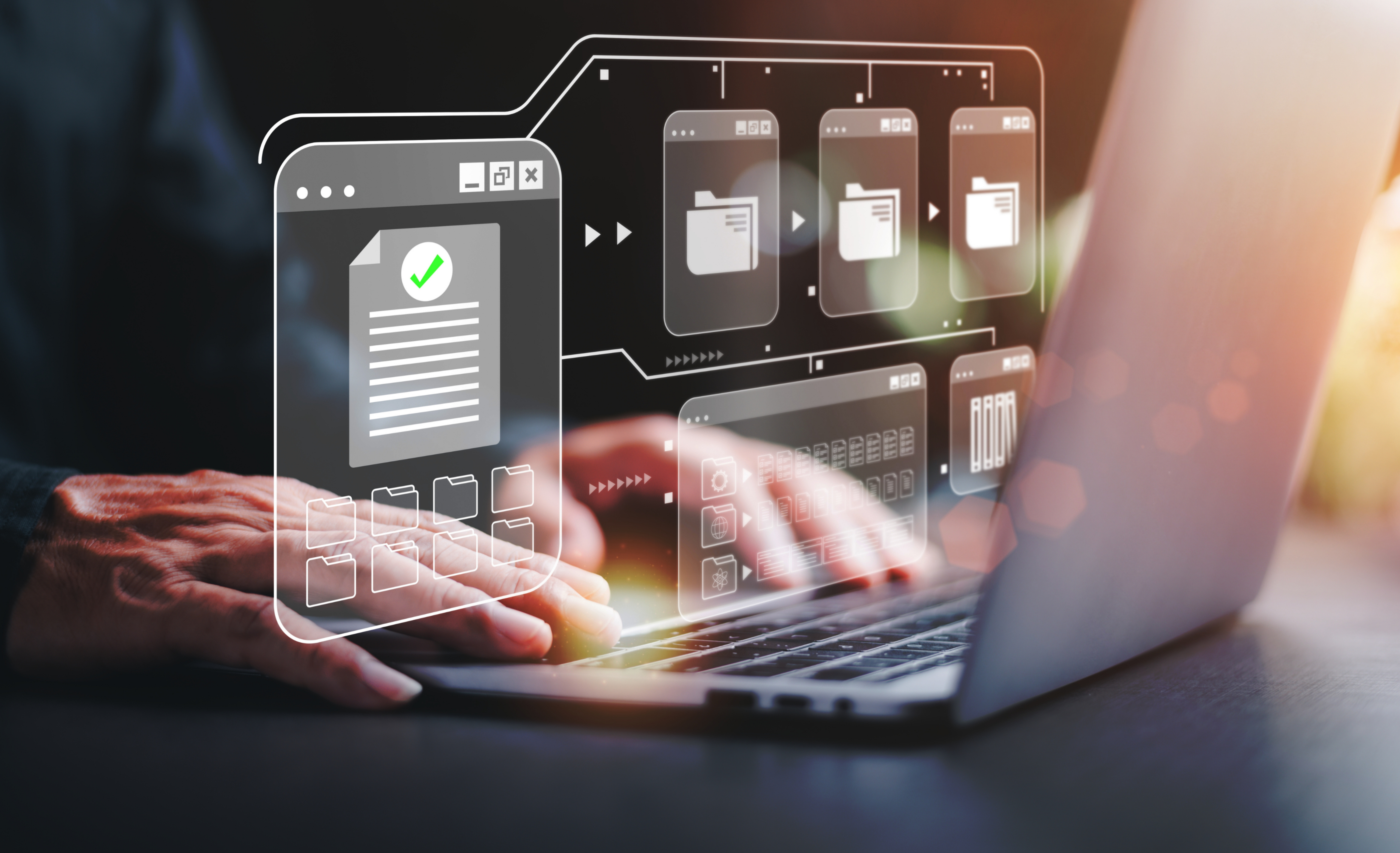
Reference & Master Data Management
Master data management (MDM) involves creating a single master record for each person, place, or thing in a business, from across internal and external data sources and applications.
This information has been de-duplicated, reconciled and enriched, becoming a consistent, reliable source. Once created, this master data serves as a trusted view of business-critical data that can be managed and shared across the business to promote accurate reporting, reduce data errors, remove redundancy, and help workers make better-informed business decisions.
Metadata Management & Data Cataloguing Solutions
Metadata management is a cross-organizational agreement on how to define informational assets for converting data into an enterprise asset.
As data volumes and diversity grow, metadata management is even more critical to derive business value from the gigantic amounts of data.Data Engineers have leveraged metadata in understanding, aggregating, grouping and sorting data for use and also, traced back many data quality problems to metadata.
Cloud Data Strategy, Execution & Migration
Akira has helped companies migrate from on-premise legacy systems to cloud based solutions by recommending appropriate cloud tools and keeping into account the usability, scalability of customers.
The company has seamlessly implemented and executed migration projects across different data capacities.
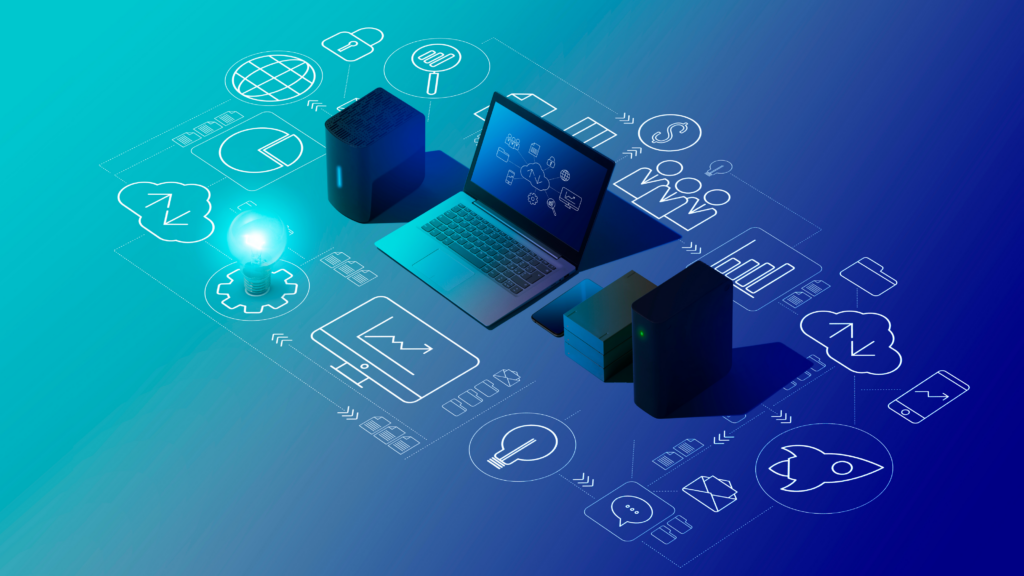
Data Virtualization Solutions
Data virtualization is an approach to integrating data from multiple sources of different types into a holistic, logical view without moving it physically.
In simple terms, data remains in original sources while users can access and analyze it virtually via special middleware.
Akira has been involved in creating from-the-scratch Data Virtualization layer for multiple clients to facilitate usgae of same in multiple BI reports.